

Evaluating estimation error is critical to interpreting biological significance and improving the ELISA microarray process. These estimates, however, are uncertain due to processing error and biological variability. Deploying ELISA in a microarray format permits simultaneous estimation of the concentrations of numerous proteins in a small sample. « lessīackground: Enzyme-linked immunosorbent assay (ELISA) is a standard immunoassay to estimate a protein's concentration in a sample. The spline method may be useful when automated prediction across simultaneous assays of numerous proteins must be applied routinely with minimal user intervention. For the 50% of the real data sets fit well by both methods, spline and logistic predictions are practically indistinguishable, varying in accuracy by less than 15%. The spline method simplifies model selection and fitting, and reliably estimates believable prediction errors. Conclusions: The spline/PCLS/MC method is a flexible, robust alternative to a logistic/NLS/propagation-of-error method to reliably predict protein concentrations and estimate their errors. Monte Carlo simulation rendered acceptable asymmetric prediction intervals for both spline and logistic models while propagation of error produced symmetric intervals that diverged unrealistically as the standard curves approached horizontal asymptotes. The relative errors of 50% of comparable spline and logistic predictions differed by less than 20%. For the real data sets, 61% of the spline predictions were more accurate than their comparable logistic predictions especially the spline predictions at the extremes of the prediction curve. Results: In contrast to the rigid logistic model, the flexible spline model gave credible fits in almost all test cases including troublesome cases with left and/or right censoring, or other asymmetries. The spline/PCLS/MC method is compared to a common method using simulated and real ELISA microarray data sets.

With MC, both modeling and measurement errors are combined to estimate prediction error. PCLS restrains the flexible spline to a fit of assay intensity more » that is a monotone function of protein concentration. Methods: We present a statistical method based on monotonic spline statistical models, penalized constrained least squares fitting (PCLS) and Monte Carlo simulation (MC) to predict concentrations and estimate prediction errors in ELISA microarray. Making sound biological inferences as well as improving the ELISA microarray process require require both concentration predictions and creditable estimates of their errors. These predictions, however, are uncertain due to processing error and biological variability.
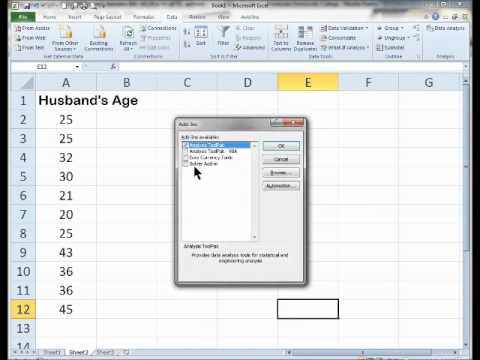
« lessīackground: A microarray of enzyme-linked immunosorbent assays, or ELISA microarray, predicts simultaneously the concentrations of numerous proteins in a small sample. Equally useful, this calibrator system can help reveal the source of the bias, thereby facilitating the elimination of the underlying problem. We demonstrate that data normalization using this system markedly reduces bias from processing factors. We developed ProMAT Calibrator, an open-source bioinformatics tool, for the rapid visualization and interpretation of the calibrator data and, if desired, data normalization. The GFP antigen is spiked into each biological sample or standard mixture and the resulting signal is more » used for calibration across chips. Specifically, we developed a sandwich ELISA for green fluorescent protein (GFP) that is included on each chip. For this reason, we have developed a calibration system that can identify and reduce systematic bias due to processing factors. Studies using antibody microarrays are susceptible to systematic bias from the various steps in the experimental process, and these biases can mask biologically significant differences. Our research group has been developing enzyme-linked immunosorbent assays (ELISA) microarray technology for the rapid and quantitative evaluation of biomarker panels.
